import pandas as pd
boc_forex_names = pd.read_csv("/Volumes/GoogleDrive/My Drive/Forecasting/bootcamp_datasets/boc_exchange/column_names.csv")
boc_forex_names
id | label | description | |
---|---|---|---|
0 | IEXE1601.CL | AUD_CLOSE | Australian dollar (close) |
1 | IEXE0301.CL | DKK_CLOSE | Danish krone (close) |
2 | EUROCAE01.CL | EUR_CLOSE | European Euro (close) |
3 | IEXE1401.CL | HKD_CLOSE | Hong Kong dollar (close) |
4 | IEXE0701.CL | JPY_CLOSE | Japanese yen (close) |
5 | IEXE2001.CL | MXN_CLOSE | Mexican peso (close) |
6 | IEXE1901.CL | NZD_CLOSE | New Zealand dollar (close) |
7 | IEXE0901.CL | NOK_CLOSE | Norwegian krone (close) |
8 | IEXE1001.CL | SEK_CLOSE | Swedish krona (close) |
9 | IEXE1101.CL | CHF_CLOSE | Swiss franc (close) |
10 | IEXE1201.CL | GBP_CLOSE | U.K. pound sterling (close) |
11 | IEXE0102 | USD_CLOSE | U.S. dollar (close) |
12 | IEXE0103 | USD_HIGH | U.S. dollar (high) |
13 | IEXE0104 | USD_LOW | U.S. dollar (low) |
14 | IEXE0106 | USD_CLOSE_90_DAY | U.S. dollar closing,90-day |
column_name_map = boc_forex_names[['id', 'label']].set_index('id').to_dict()['label']
boc_forex_df = pd.read_csv("/Volumes/GoogleDrive/My Drive/Forecasting/bootcamp_datasets/boc_exchange/LEGACY_CLOSING_RATES.csv")
boc_forex_df
date | IEXE1601.CL | IEXE0301.CL | EUROCAE01.CL | IEXE1401.CL | IEXE0701.CL | IEXE2001.CL | IEXE1901.CL | IEXE0901.CL | IEXE1001.CL | IEXE1101.CL | IEXE1201.CL | IEXE0102 | IEXE0103 | IEXE0104 | IEXE0106 | |
---|---|---|---|---|---|---|---|---|---|---|---|---|---|---|---|---|
0 | 2007-05-01 | 0.9200 | 0.2000 | 1.5100 | 0.141970 | 0.009271 | 0.10000 | 0.8200 | 0.1860 | 0.1700 | 0.9100 | 2.2199 | 1.1105 | 1.1116 | 1.1048 | 1.1075 |
1 | 2007-05-02 | 0.9100 | 0.2000 | 1.5100 | 0.141740 | 0.009232 | 0.10000 | 0.8200 | 0.1854 | 0.1700 | 0.9100 | 2.2055 | 1.1087 | 1.1115 | 1.1066 | 1.1057 |
2 | 2007-05-03 | 0.9100 | 0.2000 | 1.5000 | 0.141496 | 0.009190 | 0.10000 | 0.8100 | 0.1847 | 0.1600 | 0.9100 | 2.1999 | 1.1066 | 1.1086 | 1.1054 | 1.1036 |
3 | 2007-05-04 | 0.9100 | 0.2000 | 1.5100 | 0.141616 | 0.009218 | 0.10000 | 0.8100 | 0.1854 | 0.1600 | 0.9100 | 2.2075 | 1.1075 | 1.1077 | 1.1032 | 1.1046 |
4 | 2007-05-07 | 0.9100 | 0.2000 | 1.5000 | 0.140908 | 0.009177 | 0.10000 | 0.8100 | 0.1843 | 0.1600 | 0.9100 | 2.1957 | 1.1018 | 1.1042 | 1.1007 | 1.0988 |
... | ... | ... | ... | ... | ... | ... | ... | ... | ... | ... | ... | ... | ... | ... | ... | ... |
2499 | 2017-04-24 | 1.0218 | 0.1974 | 1.4684 | 0.173684 | 0.012310 | 0.07215 | 0.9477 | 0.1579 | 0.1525 | 1.3568 | 1.7280 | 1.3511 | 1.3521 | 1.3425 | 1.3493 |
2500 | 2017-04-25 | 1.0224 | 0.1994 | 1.4837 | 0.174374 | 0.012210 | 0.07183 | 0.9426 | 0.1586 | 0.1548 | 1.3661 | 1.7407 | 1.3565 | 1.3626 | 1.3565 | 1.3547 |
2501 | 2017-04-26 | 1.0176 | 0.1995 | 1.4847 | 0.174965 | 0.012260 | 0.07098 | 0.9382 | 0.1585 | 0.1554 | 1.3707 | 1.7493 | 1.3612 | 1.3623 | 1.3543 | 1.3594 |
2502 | 2017-04-27 | 1.0176 | 0.1992 | 1.4815 | 0.175103 | 0.012250 | 0.07151 | 0.9369 | 0.1590 | 0.1543 | 1.3704 | 1.7584 | 1.3624 | 1.3670 | 1.3574 | 1.3605 |
2503 | 2017-04-28 | 1.0222 | 0.1999 | 1.4870 | 0.175485 | 0.012250 | 0.07253 | 0.9373 | 0.1590 | 0.1541 | 1.3719 | 1.7679 | 1.3650 | 1.3697 | 1.3635 | 1.3631 |
2504 rows × 16 columns
boc_forex_df = boc_forex_df.rename(column_name_map, axis=1)
boc_forex_df = boc_forex_df[['date'] + [col for col in boc_forex_df if col.endswith('_CLOSE')]]
boc_forex_df
date | AUD_CLOSE | DKK_CLOSE | EUR_CLOSE | HKD_CLOSE | JPY_CLOSE | MXN_CLOSE | NZD_CLOSE | NOK_CLOSE | SEK_CLOSE | CHF_CLOSE | GBP_CLOSE | USD_CLOSE | |
---|---|---|---|---|---|---|---|---|---|---|---|---|---|
0 | 2007-05-01 | 0.9200 | 0.2000 | 1.5100 | 0.141970 | 0.009271 | 0.10000 | 0.8200 | 0.1860 | 0.1700 | 0.9100 | 2.2199 | 1.1105 |
1 | 2007-05-02 | 0.9100 | 0.2000 | 1.5100 | 0.141740 | 0.009232 | 0.10000 | 0.8200 | 0.1854 | 0.1700 | 0.9100 | 2.2055 | 1.1087 |
2 | 2007-05-03 | 0.9100 | 0.2000 | 1.5000 | 0.141496 | 0.009190 | 0.10000 | 0.8100 | 0.1847 | 0.1600 | 0.9100 | 2.1999 | 1.1066 |
3 | 2007-05-04 | 0.9100 | 0.2000 | 1.5100 | 0.141616 | 0.009218 | 0.10000 | 0.8100 | 0.1854 | 0.1600 | 0.9100 | 2.2075 | 1.1075 |
4 | 2007-05-07 | 0.9100 | 0.2000 | 1.5000 | 0.140908 | 0.009177 | 0.10000 | 0.8100 | 0.1843 | 0.1600 | 0.9100 | 2.1957 | 1.1018 |
... | ... | ... | ... | ... | ... | ... | ... | ... | ... | ... | ... | ... | ... |
2499 | 2017-04-24 | 1.0218 | 0.1974 | 1.4684 | 0.173684 | 0.012310 | 0.07215 | 0.9477 | 0.1579 | 0.1525 | 1.3568 | 1.7280 | 1.3511 |
2500 | 2017-04-25 | 1.0224 | 0.1994 | 1.4837 | 0.174374 | 0.012210 | 0.07183 | 0.9426 | 0.1586 | 0.1548 | 1.3661 | 1.7407 | 1.3565 |
2501 | 2017-04-26 | 1.0176 | 0.1995 | 1.4847 | 0.174965 | 0.012260 | 0.07098 | 0.9382 | 0.1585 | 0.1554 | 1.3707 | 1.7493 | 1.3612 |
2502 | 2017-04-27 | 1.0176 | 0.1992 | 1.4815 | 0.175103 | 0.012250 | 0.07151 | 0.9369 | 0.1590 | 0.1543 | 1.3704 | 1.7584 | 1.3624 |
2503 | 2017-04-28 | 1.0222 | 0.1999 | 1.4870 | 0.175485 | 0.012250 | 0.07253 | 0.9373 | 0.1590 | 0.1541 | 1.3719 | 1.7679 | 1.3650 |
2504 rows × 13 columns
boc_forex_df.plot()
<AxesSubplot:>
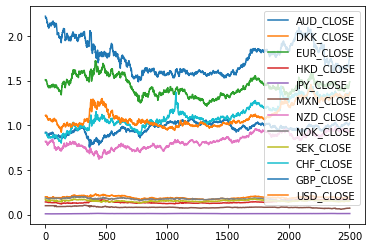
boc_forex_df.to_csv("/Volumes/GoogleDrive/My Drive/Forecasting/bootcamp_datasets/boc_exchange/dataset.csv", index=False)
pd.read_csv("/Volumes/GoogleDrive/My Drive/Forecasting/bootcamp_datasets/boc_exchange/dataset.csv")
date | AUD_CLOSE | DKK_CLOSE | EUR_CLOSE | HKD_CLOSE | JPY_CLOSE | MXN_CLOSE | NZD_CLOSE | NOK_CLOSE | SEK_CLOSE | CHF_CLOSE | GBP_CLOSE | USD_CLOSE | |
---|---|---|---|---|---|---|---|---|---|---|---|---|---|
0 | 2007-05-01 | 0.9200 | 0.2000 | 1.5100 | 0.141970 | 0.009271 | 0.10000 | 0.8200 | 0.1860 | 0.1700 | 0.9100 | 2.2199 | 1.1105 |
1 | 2007-05-02 | 0.9100 | 0.2000 | 1.5100 | 0.141740 | 0.009232 | 0.10000 | 0.8200 | 0.1854 | 0.1700 | 0.9100 | 2.2055 | 1.1087 |
2 | 2007-05-03 | 0.9100 | 0.2000 | 1.5000 | 0.141496 | 0.009190 | 0.10000 | 0.8100 | 0.1847 | 0.1600 | 0.9100 | 2.1999 | 1.1066 |
3 | 2007-05-04 | 0.9100 | 0.2000 | 1.5100 | 0.141616 | 0.009218 | 0.10000 | 0.8100 | 0.1854 | 0.1600 | 0.9100 | 2.2075 | 1.1075 |
4 | 2007-05-07 | 0.9100 | 0.2000 | 1.5000 | 0.140908 | 0.009177 | 0.10000 | 0.8100 | 0.1843 | 0.1600 | 0.9100 | 2.1957 | 1.1018 |
... | ... | ... | ... | ... | ... | ... | ... | ... | ... | ... | ... | ... | ... |
2499 | 2017-04-24 | 1.0218 | 0.1974 | 1.4684 | 0.173684 | 0.012310 | 0.07215 | 0.9477 | 0.1579 | 0.1525 | 1.3568 | 1.7280 | 1.3511 |
2500 | 2017-04-25 | 1.0224 | 0.1994 | 1.4837 | 0.174374 | 0.012210 | 0.07183 | 0.9426 | 0.1586 | 0.1548 | 1.3661 | 1.7407 | 1.3565 |
2501 | 2017-04-26 | 1.0176 | 0.1995 | 1.4847 | 0.174965 | 0.012260 | 0.07098 | 0.9382 | 0.1585 | 0.1554 | 1.3707 | 1.7493 | 1.3612 |
2502 | 2017-04-27 | 1.0176 | 0.1992 | 1.4815 | 0.175103 | 0.012250 | 0.07151 | 0.9369 | 0.1590 | 0.1543 | 1.3704 | 1.7584 | 1.3624 |
2503 | 2017-04-28 | 1.0222 | 0.1999 | 1.4870 | 0.175485 | 0.012250 | 0.07253 | 0.9373 | 0.1590 | 0.1541 | 1.3719 | 1.7679 | 1.3650 |
2504 rows × 13 columns