M5 Data Preparation#
This notebook helps with loading and transforming the M5 dataset into higher-level, aggregate time series. M5, by default, is provided as a set of 30,490 individual time series. These correspond to individual product sales figures at individual Walmart stores. These time series can be naturally aggregated according to a hierarchy:
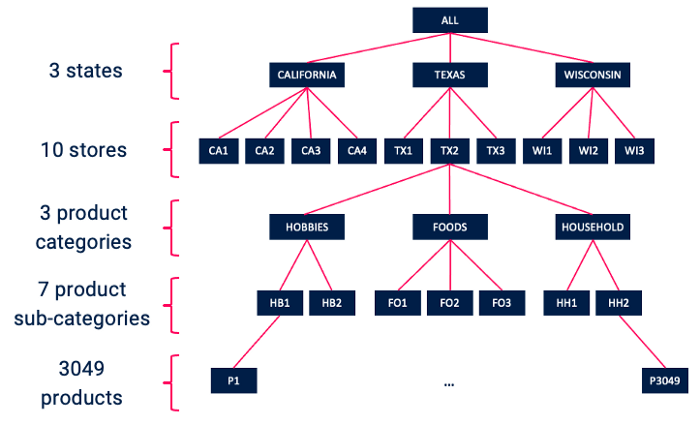
As we can see, the 30,490 time series correspond to sales figures for 3049 individual products across 10 stores. The products each belong to one of three product categories (Hobbies, Foods, and Household), which can be further broken down into a total of 7 product subcategories. The 10 stores each belong to one of three states (California, Texas, and Wisconsin).
Other information are also provided as part of the M5 dataset, including product sell prices and a calendar of important events (e.g. holidays, relevant dates for nutrition assistance programs).
The following code has been adapted from this repository for the official 2nd place M5 solution.
Load data#
import pandas as pd
m5_input_path = "/ssd003/projects/forecasting_bootcamp/bootcamp_datasets/m5-forecasting-accuracy"
sell_price = pd.read_csv(f'{m5_input_path}/sell_prices.csv')
calendar = pd.read_csv(f'{m5_input_path}/calendar.csv')
train = pd.read_csv(f'{m5_input_path}/sales_train_evaluation.csv').set_index('id')
sample_sub = pd.read_csv(f'{m5_input_path}/sample_submission.csv')
Build aggregate dataset#
# Get column groups
cat_cols = ['item_id', 'dept_id', 'cat_id', 'store_id', 'state_id']
ts_cols = [col for col in train.columns if col not in cat_cols]
ts_dict = {t: int(t[2:]) for t in ts_cols}
# Describe data
print(' unique forecasts: %i' % train.shape[0])
for col in cat_cols:
print(' N_unique %s: %i' % (col, train[col].nunique()))
unique forecasts: 30490
N_unique item_id: 3049
N_unique dept_id: 7
N_unique cat_id: 3
N_unique store_id: 10
N_unique state_id: 3
%%time
# 1. All products, all stores, all states (1 series)
all_sales = pd.DataFrame(train[ts_cols].sum()).transpose()
all_sales['id_str'] = 'all'
all_sales = all_sales[ ['id_str'] + [c for c in all_sales if c not in ['id_str']] ]
all_sales
CPU times: user 133 ms, sys: 140 ms, total: 273 ms
Wall time: 269 ms
id_str | d_1 | d_2 | d_3 | d_4 | d_5 | d_6 | d_7 | d_8 | d_9 | ... | d_1932 | d_1933 | d_1934 | d_1935 | d_1936 | d_1937 | d_1938 | d_1939 | d_1940 | d_1941 | |
---|---|---|---|---|---|---|---|---|---|---|---|---|---|---|---|---|---|---|---|---|---|
0 | all | 32631 | 31749 | 23783 | 25412 | 19146 | 29211 | 28010 | 37932 | 32736 | ... | 44073 | 54308 | 59921 | 42362 | 38777 | 37096 | 36963 | 42552 | 51518 | 54338 |
1 rows × 1942 columns
%%time
# 2. All products by state (3 series)
state_sales = train.groupby('state_id',as_index=False)[ts_cols].sum()
state_sales['id_str'] = state_sales['state_id']
state_sales = state_sales[ ['id_str'] + [c for c in state_sales if c not in ['id_str']] ]
state_sales = state_sales.drop(['state_id'],axis=1)
state_sales
CPU times: user 734 ms, sys: 813 ms, total: 1.55 s
Wall time: 1.58 s
id_str | d_1 | d_2 | d_3 | d_4 | d_5 | d_6 | d_7 | d_8 | d_9 | ... | d_1932 | d_1933 | d_1934 | d_1935 | d_1936 | d_1937 | d_1938 | d_1939 | d_1940 | d_1941 | |
---|---|---|---|---|---|---|---|---|---|---|---|---|---|---|---|---|---|---|---|---|---|
0 | CA | 14195 | 13805 | 10108 | 11047 | 9925 | 11322 | 12251 | 16610 | 14696 | ... | 18471 | 23037 | 24704 | 17721 | 16150 | 15678 | 16297 | 17430 | 23103 | 24644 |
1 | TX | 9438 | 9630 | 6778 | 7381 | 5912 | 9006 | 6226 | 9440 | 9376 | ... | 12320 | 12366 | 16249 | 12228 | 11370 | 10375 | 9162 | 12303 | 13681 | 14815 |
2 | WI | 8998 | 8314 | 6897 | 6984 | 3309 | 8883 | 9533 | 11882 | 8664 | ... | 13282 | 18905 | 18968 | 12413 | 11257 | 11043 | 11504 | 12819 | 14734 | 14879 |
3 rows × 1942 columns
%%time
# 3. All products by store (10 series)
store_sales = train.groupby('store_id',as_index=False)[ts_cols].sum()
store_sales['id_str'] = store_sales['store_id']
store_sales = store_sales[ ['id_str'] + [c for c in store_sales if c not in ['id_str']] ]
store_sales = store_sales.drop(['store_id'],axis=1)
store_sales
CPU times: user 735 ms, sys: 1.87 s, total: 2.61 s
Wall time: 2.6 s
id_str | d_1 | d_2 | d_3 | d_4 | d_5 | d_6 | d_7 | d_8 | d_9 | ... | d_1932 | d_1933 | d_1934 | d_1935 | d_1936 | d_1937 | d_1938 | d_1939 | d_1940 | d_1941 | |
---|---|---|---|---|---|---|---|---|---|---|---|---|---|---|---|---|---|---|---|---|---|
0 | CA_1 | 4337 | 4155 | 2816 | 3051 | 2630 | 3276 | 3450 | 5437 | 4340 | ... | 4951 | 6245 | 6707 | 4568 | 3949 | 3995 | 4136 | 4433 | 5764 | 6289 |
1 | CA_2 | 3494 | 3046 | 2121 | 2324 | 1942 | 2288 | 2629 | 3729 | 2957 | ... | 4839 | 6507 | 6710 | 4238 | 3963 | 3789 | 4037 | 4751 | 7120 | 6614 |
2 | CA_3 | 4739 | 4827 | 3785 | 4232 | 3817 | 4369 | 4703 | 5456 | 5581 | ... | 6046 | 7029 | 7969 | 5891 | 5523 | 5375 | 5580 | 5542 | 7073 | 8144 |
3 | CA_4 | 1625 | 1777 | 1386 | 1440 | 1536 | 1389 | 1469 | 1988 | 1818 | ... | 2635 | 3256 | 3318 | 3024 | 2715 | 2519 | 2544 | 2704 | 3146 | 3597 |
4 | TX_1 | 2556 | 2687 | 1822 | 2258 | 1694 | 2734 | 1691 | 2820 | 2887 | ... | 3610 | 3787 | 5000 | 3708 | 3310 | 3147 | 2748 | 3664 | 4167 | 4624 |
5 | TX_2 | 3852 | 3937 | 2731 | 2954 | 2492 | 3439 | 2588 | 3772 | 3657 | ... | 4415 | 4424 | 5866 | 4179 | 4008 | 3518 | 3126 | 4249 | 4802 | 5217 |
6 | TX_3 | 3030 | 3006 | 2225 | 2169 | 1726 | 2833 | 1947 | 2848 | 2832 | ... | 4295 | 4155 | 5383 | 4341 | 4052 | 3710 | 3288 | 4390 | 4712 | 4974 |
7 | WI_1 | 2704 | 2194 | 1562 | 1251 | 2 | 2049 | 2815 | 3248 | 1674 | ... | 3978 | 5527 | 5488 | 3505 | 3323 | 3242 | 3478 | 3813 | 5002 | 5054 |
8 | WI_2 | 2256 | 1922 | 2018 | 2522 | 1175 | 2244 | 2232 | 2643 | 2140 | ... | 5317 | 7704 | 7586 | 5011 | 4628 | 4533 | 4628 | 4880 | 5213 | 5068 |
9 | WI_3 | 4038 | 4198 | 3317 | 3211 | 2132 | 4590 | 4486 | 5991 | 4850 | ... | 3987 | 5674 | 5894 | 3897 | 3306 | 3268 | 3398 | 4126 | 4519 | 4757 |
10 rows × 1942 columns
%%time
# 4. All products by category (3 series)
cat_sales = train.groupby('cat_id',as_index=False)[ts_cols].sum()
cat_sales['id_str'] = cat_sales['cat_id']
cat_sales = cat_sales[ ['id_str'] + [c for c in cat_sales if c not in ['id_str']] ]
cat_sales = cat_sales.drop(['cat_id'],axis=1)
cat_sales
CPU times: user 725 ms, sys: 279 ms, total: 1 s
Wall time: 1 s
id_str | d_1 | d_2 | d_3 | d_4 | d_5 | d_6 | d_7 | d_8 | d_9 | ... | d_1932 | d_1933 | d_1934 | d_1935 | d_1936 | d_1937 | d_1938 | d_1939 | d_1940 | d_1941 | |
---|---|---|---|---|---|---|---|---|---|---|---|---|---|---|---|---|---|---|---|---|---|
0 | FOODS | 23178 | 22758 | 17174 | 18878 | 14603 | 22093 | 20490 | 27751 | 24862 | ... | 30107 | 36576 | 41447 | 29158 | 26148 | 24790 | 24737 | 28136 | 33599 | 35967 |
1 | HOBBIES | 3764 | 3357 | 2682 | 2669 | 1814 | 3220 | 2944 | 3986 | 2899 | ... | 3871 | 4893 | 5016 | 3528 | 3629 | 3740 | 3475 | 4143 | 5333 | 5280 |
2 | HOUSEHOLD | 5689 | 5634 | 3927 | 3865 | 2729 | 3898 | 4576 | 6195 | 4975 | ... | 10095 | 12839 | 13458 | 9676 | 9000 | 8566 | 8751 | 10273 | 12586 | 13091 |
3 rows × 1942 columns
%%time
# 5. All products by department (7 series)
dept_sales = train.groupby('dept_id',as_index=False)[ts_cols].sum()
dept_sales['id_str'] = dept_sales['dept_id']
dept_sales = dept_sales[ ['id_str'] + [c for c in dept_sales if c not in ['id_str']] ]
dept_sales = dept_sales.drop(['dept_id'],axis=1)
dept_sales
CPU times: user 722 ms, sys: 491 ms, total: 1.21 s
Wall time: 1.21 s
id_str | d_1 | d_2 | d_3 | d_4 | d_5 | d_6 | d_7 | d_8 | d_9 | ... | d_1932 | d_1933 | d_1934 | d_1935 | d_1936 | d_1937 | d_1938 | d_1939 | d_1940 | d_1941 | |
---|---|---|---|---|---|---|---|---|---|---|---|---|---|---|---|---|---|---|---|---|---|
0 | FOODS_1 | 2343 | 2216 | 1657 | 1508 | 1209 | 1897 | 1903 | 2235 | 1925 | ... | 4130 | 4257 | 4225 | 3264 | 3119 | 3073 | 3251 | 3735 | 4195 | 4031 |
1 | FOODS_2 | 4094 | 4209 | 3174 | 3606 | 2869 | 4375 | 3349 | 4384 | 4273 | ... | 5842 | 7563 | 9069 | 6226 | 5311 | 4648 | 4551 | 4815 | 5940 | 6535 |
2 | FOODS_3 | 16741 | 16333 | 12343 | 13764 | 10525 | 15821 | 15238 | 21132 | 18664 | ... | 20135 | 24756 | 28153 | 19668 | 17718 | 17069 | 16935 | 19586 | 23464 | 25401 |
3 | HOBBIES_1 | 3610 | 3172 | 2497 | 2531 | 1714 | 3133 | 2855 | 3831 | 2741 | ... | 3422 | 4414 | 4464 | 3109 | 3142 | 3291 | 3027 | 3761 | 4832 | 4720 |
4 | HOBBIES_2 | 154 | 185 | 185 | 138 | 100 | 87 | 89 | 155 | 158 | ... | 449 | 479 | 552 | 419 | 487 | 449 | 448 | 382 | 501 | 560 |
5 | HOUSEHOLD_1 | 4105 | 3858 | 2827 | 2732 | 1802 | 2664 | 3161 | 4334 | 3494 | ... | 7936 | 10042 | 10572 | 7609 | 6999 | 6641 | 6759 | 7974 | 9668 | 10165 |
6 | HOUSEHOLD_2 | 1584 | 1776 | 1100 | 1133 | 927 | 1234 | 1415 | 1861 | 1481 | ... | 2159 | 2797 | 2886 | 2067 | 2001 | 1925 | 1992 | 2299 | 2918 | 2926 |
7 rows × 1942 columns
## TOP LEVEL aggregates + TOTAL
all_aggregates = pd.concat([all_sales,state_sales,store_sales,cat_sales,dept_sales],ignore_index=True)
all_aggregates
id_str | d_1 | d_2 | d_3 | d_4 | d_5 | d_6 | d_7 | d_8 | d_9 | ... | d_1932 | d_1933 | d_1934 | d_1935 | d_1936 | d_1937 | d_1938 | d_1939 | d_1940 | d_1941 | |
---|---|---|---|---|---|---|---|---|---|---|---|---|---|---|---|---|---|---|---|---|---|
0 | all | 32631 | 31749 | 23783 | 25412 | 19146 | 29211 | 28010 | 37932 | 32736 | ... | 44073 | 54308 | 59921 | 42362 | 38777 | 37096 | 36963 | 42552 | 51518 | 54338 |
1 | CA | 14195 | 13805 | 10108 | 11047 | 9925 | 11322 | 12251 | 16610 | 14696 | ... | 18471 | 23037 | 24704 | 17721 | 16150 | 15678 | 16297 | 17430 | 23103 | 24644 |
2 | TX | 9438 | 9630 | 6778 | 7381 | 5912 | 9006 | 6226 | 9440 | 9376 | ... | 12320 | 12366 | 16249 | 12228 | 11370 | 10375 | 9162 | 12303 | 13681 | 14815 |
3 | WI | 8998 | 8314 | 6897 | 6984 | 3309 | 8883 | 9533 | 11882 | 8664 | ... | 13282 | 18905 | 18968 | 12413 | 11257 | 11043 | 11504 | 12819 | 14734 | 14879 |
4 | CA_1 | 4337 | 4155 | 2816 | 3051 | 2630 | 3276 | 3450 | 5437 | 4340 | ... | 4951 | 6245 | 6707 | 4568 | 3949 | 3995 | 4136 | 4433 | 5764 | 6289 |
5 | CA_2 | 3494 | 3046 | 2121 | 2324 | 1942 | 2288 | 2629 | 3729 | 2957 | ... | 4839 | 6507 | 6710 | 4238 | 3963 | 3789 | 4037 | 4751 | 7120 | 6614 |
6 | CA_3 | 4739 | 4827 | 3785 | 4232 | 3817 | 4369 | 4703 | 5456 | 5581 | ... | 6046 | 7029 | 7969 | 5891 | 5523 | 5375 | 5580 | 5542 | 7073 | 8144 |
7 | CA_4 | 1625 | 1777 | 1386 | 1440 | 1536 | 1389 | 1469 | 1988 | 1818 | ... | 2635 | 3256 | 3318 | 3024 | 2715 | 2519 | 2544 | 2704 | 3146 | 3597 |
8 | TX_1 | 2556 | 2687 | 1822 | 2258 | 1694 | 2734 | 1691 | 2820 | 2887 | ... | 3610 | 3787 | 5000 | 3708 | 3310 | 3147 | 2748 | 3664 | 4167 | 4624 |
9 | TX_2 | 3852 | 3937 | 2731 | 2954 | 2492 | 3439 | 2588 | 3772 | 3657 | ... | 4415 | 4424 | 5866 | 4179 | 4008 | 3518 | 3126 | 4249 | 4802 | 5217 |
10 | TX_3 | 3030 | 3006 | 2225 | 2169 | 1726 | 2833 | 1947 | 2848 | 2832 | ... | 4295 | 4155 | 5383 | 4341 | 4052 | 3710 | 3288 | 4390 | 4712 | 4974 |
11 | WI_1 | 2704 | 2194 | 1562 | 1251 | 2 | 2049 | 2815 | 3248 | 1674 | ... | 3978 | 5527 | 5488 | 3505 | 3323 | 3242 | 3478 | 3813 | 5002 | 5054 |
12 | WI_2 | 2256 | 1922 | 2018 | 2522 | 1175 | 2244 | 2232 | 2643 | 2140 | ... | 5317 | 7704 | 7586 | 5011 | 4628 | 4533 | 4628 | 4880 | 5213 | 5068 |
13 | WI_3 | 4038 | 4198 | 3317 | 3211 | 2132 | 4590 | 4486 | 5991 | 4850 | ... | 3987 | 5674 | 5894 | 3897 | 3306 | 3268 | 3398 | 4126 | 4519 | 4757 |
14 | FOODS | 23178 | 22758 | 17174 | 18878 | 14603 | 22093 | 20490 | 27751 | 24862 | ... | 30107 | 36576 | 41447 | 29158 | 26148 | 24790 | 24737 | 28136 | 33599 | 35967 |
15 | HOBBIES | 3764 | 3357 | 2682 | 2669 | 1814 | 3220 | 2944 | 3986 | 2899 | ... | 3871 | 4893 | 5016 | 3528 | 3629 | 3740 | 3475 | 4143 | 5333 | 5280 |
16 | HOUSEHOLD | 5689 | 5634 | 3927 | 3865 | 2729 | 3898 | 4576 | 6195 | 4975 | ... | 10095 | 12839 | 13458 | 9676 | 9000 | 8566 | 8751 | 10273 | 12586 | 13091 |
17 | FOODS_1 | 2343 | 2216 | 1657 | 1508 | 1209 | 1897 | 1903 | 2235 | 1925 | ... | 4130 | 4257 | 4225 | 3264 | 3119 | 3073 | 3251 | 3735 | 4195 | 4031 |
18 | FOODS_2 | 4094 | 4209 | 3174 | 3606 | 2869 | 4375 | 3349 | 4384 | 4273 | ... | 5842 | 7563 | 9069 | 6226 | 5311 | 4648 | 4551 | 4815 | 5940 | 6535 |
19 | FOODS_3 | 16741 | 16333 | 12343 | 13764 | 10525 | 15821 | 15238 | 21132 | 18664 | ... | 20135 | 24756 | 28153 | 19668 | 17718 | 17069 | 16935 | 19586 | 23464 | 25401 |
20 | HOBBIES_1 | 3610 | 3172 | 2497 | 2531 | 1714 | 3133 | 2855 | 3831 | 2741 | ... | 3422 | 4414 | 4464 | 3109 | 3142 | 3291 | 3027 | 3761 | 4832 | 4720 |
21 | HOBBIES_2 | 154 | 185 | 185 | 138 | 100 | 87 | 89 | 155 | 158 | ... | 449 | 479 | 552 | 419 | 487 | 449 | 448 | 382 | 501 | 560 |
22 | HOUSEHOLD_1 | 4105 | 3858 | 2827 | 2732 | 1802 | 2664 | 3161 | 4334 | 3494 | ... | 7936 | 10042 | 10572 | 7609 | 6999 | 6641 | 6759 | 7974 | 9668 | 10165 |
23 | HOUSEHOLD_2 | 1584 | 1776 | 1100 | 1133 | 927 | 1234 | 1415 | 1861 | 1481 | ... | 2159 | 2797 | 2886 | 2067 | 2001 | 1925 | 1992 | 2299 | 2918 | 2926 |
24 rows × 1942 columns
main_df = all_aggregates.set_index('id_str').T
main_df['date'] = calendar[['d', 'date']].set_index('d')['date']
main_df = main_df.set_index('date')
main_df.index = pd.DatetimeIndex(main_df.index)
main_df
id_str | all | CA | TX | WI | CA_1 | CA_2 | CA_3 | CA_4 | TX_1 | TX_2 | ... | FOODS | HOBBIES | HOUSEHOLD | FOODS_1 | FOODS_2 | FOODS_3 | HOBBIES_1 | HOBBIES_2 | HOUSEHOLD_1 | HOUSEHOLD_2 |
---|---|---|---|---|---|---|---|---|---|---|---|---|---|---|---|---|---|---|---|---|---|
date | |||||||||||||||||||||
2011-01-29 | 32631 | 14195 | 9438 | 8998 | 4337 | 3494 | 4739 | 1625 | 2556 | 3852 | ... | 23178 | 3764 | 5689 | 2343 | 4094 | 16741 | 3610 | 154 | 4105 | 1584 |
2011-01-30 | 31749 | 13805 | 9630 | 8314 | 4155 | 3046 | 4827 | 1777 | 2687 | 3937 | ... | 22758 | 3357 | 5634 | 2216 | 4209 | 16333 | 3172 | 185 | 3858 | 1776 |
2011-01-31 | 23783 | 10108 | 6778 | 6897 | 2816 | 2121 | 3785 | 1386 | 1822 | 2731 | ... | 17174 | 2682 | 3927 | 1657 | 3174 | 12343 | 2497 | 185 | 2827 | 1100 |
2011-02-01 | 25412 | 11047 | 7381 | 6984 | 3051 | 2324 | 4232 | 1440 | 2258 | 2954 | ... | 18878 | 2669 | 3865 | 1508 | 3606 | 13764 | 2531 | 138 | 2732 | 1133 |
2011-02-02 | 19146 | 9925 | 5912 | 3309 | 2630 | 1942 | 3817 | 1536 | 1694 | 2492 | ... | 14603 | 1814 | 2729 | 1209 | 2869 | 10525 | 1714 | 100 | 1802 | 927 |
... | ... | ... | ... | ... | ... | ... | ... | ... | ... | ... | ... | ... | ... | ... | ... | ... | ... | ... | ... | ... | ... |
2016-05-18 | 37096 | 15678 | 10375 | 11043 | 3995 | 3789 | 5375 | 2519 | 3147 | 3518 | ... | 24790 | 3740 | 8566 | 3073 | 4648 | 17069 | 3291 | 449 | 6641 | 1925 |
2016-05-19 | 36963 | 16297 | 9162 | 11504 | 4136 | 4037 | 5580 | 2544 | 2748 | 3126 | ... | 24737 | 3475 | 8751 | 3251 | 4551 | 16935 | 3027 | 448 | 6759 | 1992 |
2016-05-20 | 42552 | 17430 | 12303 | 12819 | 4433 | 4751 | 5542 | 2704 | 3664 | 4249 | ... | 28136 | 4143 | 10273 | 3735 | 4815 | 19586 | 3761 | 382 | 7974 | 2299 |
2016-05-21 | 51518 | 23103 | 13681 | 14734 | 5764 | 7120 | 7073 | 3146 | 4167 | 4802 | ... | 33599 | 5333 | 12586 | 4195 | 5940 | 23464 | 4832 | 501 | 9668 | 2918 |
2016-05-22 | 54338 | 24644 | 14815 | 14879 | 6289 | 6614 | 8144 | 3597 | 4624 | 5217 | ... | 35967 | 5280 | 13091 | 4031 | 6535 | 25401 | 4720 | 560 | 10165 | 2926 |
1941 rows × 24 columns
Aggregate time series visualization#
Visualize data using different aggregation levels, e.g. by product category and by state. Scale the data to help vizualize differences in patterns.
import matplotlib.pyplot as plt
from sklearn.preprocessing import scale
fig, ax = plt.subplots(figsize=(15,4))
ax.plot(main_df.index, scale(main_df['HOBBIES']), label='HOBBIES', alpha=0.5)
ax.plot(main_df.index, scale(main_df['FOODS']), label='FOODS', alpha=0.5)
ax.plot(main_df.index, scale(main_df['HOUSEHOLD']), label='HOUSEHOLD', alpha=0.5)
plt.legend()
plt.title("Total Sales By Product Category")
Text(0.5, 1.0, 'Total Sales By Product Category')
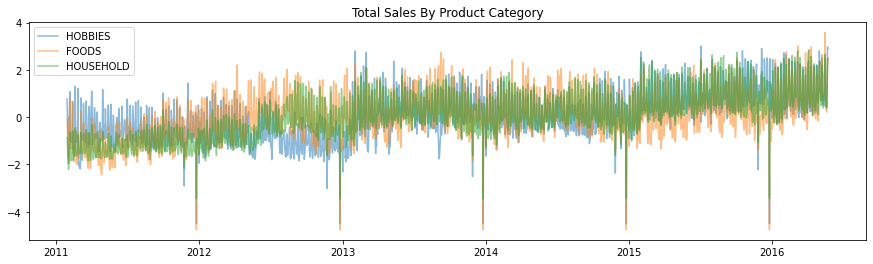
fig, ax = plt.subplots(figsize=(15,4))
ax.plot(main_df.index, scale(main_df['CA']), label='CA', alpha=0.5)
ax.plot(main_df.index, scale(main_df['TX']), label='TX', alpha=0.5)
ax.plot(main_df.index, scale(main_df['WI']), label='WI', alpha=0.5)
plt.legend()
plt.title("Total Sales By State")
Text(0.5, 1.0, 'Total Sales By State')
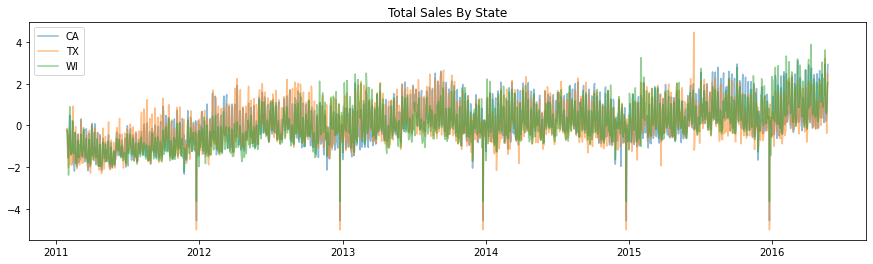
Visualize individual product sales time series.#
product_sales = train[ts_cols].T
product_sales['date'] = calendar[['d', 'date']].set_index('d')['date']
product_sales = product_sales.set_index('date')
product_sales.index = pd.DatetimeIndex(product_sales.index)
product_sales
id | HOBBIES_1_001_CA_1_evaluation | HOBBIES_1_002_CA_1_evaluation | HOBBIES_1_003_CA_1_evaluation | HOBBIES_1_004_CA_1_evaluation | HOBBIES_1_005_CA_1_evaluation | HOBBIES_1_006_CA_1_evaluation | HOBBIES_1_007_CA_1_evaluation | HOBBIES_1_008_CA_1_evaluation | HOBBIES_1_009_CA_1_evaluation | HOBBIES_1_010_CA_1_evaluation | ... | FOODS_3_818_WI_3_evaluation | FOODS_3_819_WI_3_evaluation | FOODS_3_820_WI_3_evaluation | FOODS_3_821_WI_3_evaluation | FOODS_3_822_WI_3_evaluation | FOODS_3_823_WI_3_evaluation | FOODS_3_824_WI_3_evaluation | FOODS_3_825_WI_3_evaluation | FOODS_3_826_WI_3_evaluation | FOODS_3_827_WI_3_evaluation |
---|---|---|---|---|---|---|---|---|---|---|---|---|---|---|---|---|---|---|---|---|---|
date | |||||||||||||||||||||
2011-01-29 | 0 | 0 | 0 | 0 | 0 | 0 | 0 | 12 | 2 | 0 | ... | 0 | 14 | 1 | 0 | 4 | 0 | 0 | 0 | 0 | 0 |
2011-01-30 | 0 | 0 | 0 | 0 | 0 | 0 | 0 | 15 | 0 | 0 | ... | 0 | 11 | 1 | 0 | 4 | 0 | 0 | 6 | 0 | 0 |
2011-01-31 | 0 | 0 | 0 | 0 | 0 | 0 | 0 | 0 | 7 | 1 | ... | 0 | 5 | 1 | 0 | 2 | 2 | 0 | 0 | 0 | 0 |
2011-02-01 | 0 | 0 | 0 | 0 | 0 | 0 | 0 | 0 | 3 | 0 | ... | 0 | 6 | 1 | 0 | 5 | 2 | 0 | 2 | 0 | 0 |
2011-02-02 | 0 | 0 | 0 | 0 | 0 | 0 | 0 | 0 | 0 | 0 | ... | 0 | 5 | 1 | 0 | 2 | 0 | 0 | 2 | 0 | 0 |
... | ... | ... | ... | ... | ... | ... | ... | ... | ... | ... | ... | ... | ... | ... | ... | ... | ... | ... | ... | ... | ... |
2016-05-18 | 0 | 0 | 0 | 1 | 0 | 0 | 1 | 5 | 0 | 1 | ... | 3 | 6 | 3 | 0 | 0 | 1 | 0 | 1 | 0 | 0 |
2016-05-19 | 3 | 0 | 2 | 3 | 0 | 0 | 0 | 4 | 0 | 1 | ... | 1 | 4 | 3 | 1 | 2 | 0 | 1 | 0 | 1 | 2 |
2016-05-20 | 3 | 0 | 3 | 0 | 2 | 5 | 1 | 1 | 0 | 0 | ... | 3 | 4 | 3 | 1 | 1 | 0 | 0 | 1 | 1 | 2 |
2016-05-21 | 0 | 0 | 0 | 2 | 1 | 2 | 1 | 40 | 1 | 0 | ... | 0 | 1 | 0 | 0 | 3 | 1 | 1 | 0 | 1 | 5 |
2016-05-22 | 1 | 0 | 1 | 6 | 0 | 0 | 0 | 32 | 0 | 1 | ... | 0 | 1 | 1 | 4 | 4 | 1 | 0 | 2 | 0 | 1 |
1941 rows × 30490 columns
product_sales['HOBBIES_1_001_CA_1_evaluation'].plot(figsize=(15,4))
<AxesSubplot:xlabel='date'>
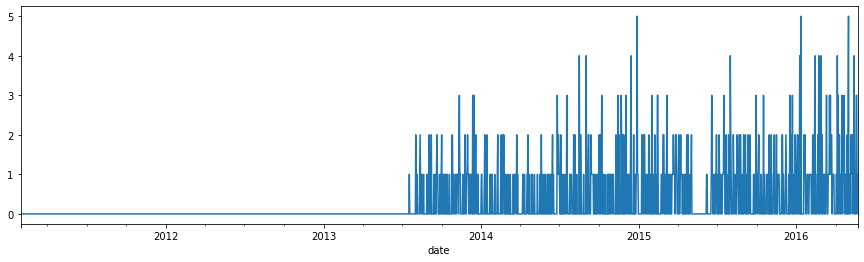
product_sales['FOODS_3_827_WI_3_evaluation'].plot(figsize=(15,4))
<AxesSubplot:xlabel='date'>
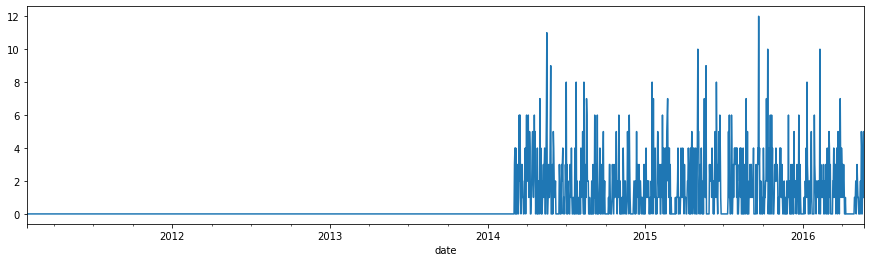